Iterative Fast 1D Forvard DCT






4.14/5 (7 votes)
Algorithm of fast forward DCT in 1D by only iteration and without branching of code
Introduction
Discret cosine’s transformation (DCT) is a well known transformation. It has very wide implementation and is used for compression, processing, e.g., due to real character of coefficients. DCT is used more often than Fourier transform. But for DCT, only one fast algorithm is well known - for 2D processing. For 1D, there is the Byeong Lee’s DCT algorithm. But its algorithm is recursive. Using it is useless for some type of equipments (e.g., GPGPU). For this target, I have created an iterative algorithm, without recursive calling and almost unlimited input data (only power of 2).
Background
For base was chosen Byeong Lee’s DCT, that is shown in the figure. The algorithm is based on Butterfly distribution. This algorithm is used wide for hardware implementation.
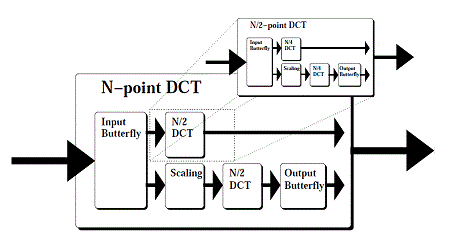
Using the Code
The developed code is based on two Paths: input with Input Butterfly and output with Output Butterfly.
Massive for keeping data between iterations is one, and allocated from heap by demand. Other massive is used for storing computed cosine’s coefficient. Every iteration divides the Front buffer into two parts: Even and Odd. After copying them into Back, the Front and Back buffers are exchanged.
//
double *Front, *Back, *temp; // Pointers on Front, Back, and temp massives of iteration.
double *Array = new double[N<<1]; // Massive with doubling size.
double *Curent_Cos = new double[N>>1]; // store for precomputing.
Front = Array; // Front pointed on begin Massive.
step = 0;
Back = Front + N; // Back pointed on center of Massive.
for(i = 0; i < N; i++)
{
Front[i] = block[i]; // Load input Data
}
while(j > 1)// Cycle of iterations Input Butterfly
{
half_N = half_N >> 1;
current_PI_half_By_N = PI_half / (double)prev_half_N;
current_PI_By_N = 0;
step_Phase = current_PI_half_By_N * 2.0;
for(i = 0; i < half_N; i++)
{
//Precompute Cosine's coefficients
Curent_Cos[i] = 0.5 / cos(current_PI_By_N + current_PI_half_By_N);
current_PI_By_N += step_Phase;
}
shif_Block = 0;
for(int x = 0; x < step; x++)
{
for(i= 0; i<half_N; i++)
{
shift = shif_Block + prev_half_N - i - 1;
Back[shif_Block + i] = Front[shif_Block + i] + Front[shift];
Back[shif_Block + half_N + i] =
(Front[shif_Block + i] - Front[shift]) * Curent_Cos[i];
}
shif_Block += prev_half_N;
}
temp = Front;
Front = Back;
Back = temp;
j = j >> 1;
step = step << 1;
prev_half_N = half_N;
}
while(j < N) // Cycle of Out ButterFly
{
shif_Block = 0;
for(int x = 0; x < step; x++)
{
for(i = 0; i<half_N - 1; i++)
{
Back[shif_Block + (i << 1)] = Front[shif_Block + i];
Back[shif_Block + (i << 1) + 1] =
Front[shif_Block + half_N + i] + Front[shif_Block + half_N + i + 1];
}
Back[shif_Block + ((half_N - 1) << 1)] = Front[shif_Block + half_N - 1];
Back[shif_Block + (half_N << 1) - 1] = Front[shif_Block + (half_N << 1) - 1];
shif_Block += prev_half_N;
}
temp = Front;
Front = Back;
Back = temp;
j = j << 1;
step = step >> 1;
half_N = prev_half_N;
prev_half_N = prev_half_N << 1;
}
for(int i = 0; i < N; i++)
{
block[i] = Front[i]; // Unload DCT coefficients
}
block[0] = block[0] / dN; // Compute DC.
Points of Interest
A very important fact is that after the first iteration, we have only one group of odd coefficients, but after the second iteration, we have two groups of odd coefficients. For those two groups, you will need identical cosine's coefficients. Store for precomputing allows to compute that coefficient only for one group. For another group will be used data from store. It's very useful for computing 1024 and more points.
In the end, I can say that the developed algorithm has more precision than the usual implementation (due to less computing of cosine's coefficient).
History
- 27th Jan, 2011: Initial post